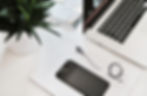
Prototype-guided Attribute-wise Interpretable Scheme for Clothing Matching
Abstract
Recently, as an essential part of people's daily life, clothing matching has gained increasing research attention. Most existing efforts focus on the numerical compatibility modeling between fashion items with advanced neural networks, and hence suffer from the poor interpretation, which makes them less applicable in real world applications. In fact, people prefer to know not only whether the given fashion items are compatible, but also the reasonable interpretations as well as advices regarding how to make the incompatible outfit harmonious. Considering that the research line of the comprehensively interpretable clothing matching is largely untapped, in this work, we propose a prototype-guided attribute-wise interpretable compatibility modeling (PAICM) scheme, which seamlessly integrates the latent compatible/incompatible prototype learning and compatibility modeling with the Bayesian personalized ranking (BPR) framework. In particular, the latent attribute interaction prototypes, learned by the non-negative matrix factorization (NMF), are treated as templates to interpret the discordant attribute and suggest the alternative item for each fashion item pair. Extensive experiments on the real-world dataset have demonstrated the effectiveness of our scheme.
Contribution
-
To the best of our knowledge, this is the first attempt to comprehensively fulfil the automatic clothing matching task by answering the three essential questions of the compatibility determination, discordant component interpretation, and alternative item suggestion.
-
We propose a prototype-guided attribute-wise interpretable compatibility modeling scheme PAICM, where the latent compatible and incompatible prototype learning and compatibility modeling is jointly regularized.
-
Extensive experiments have been conducted on the real-world dataset, which demonstrates the effectiveness of the proposed scheme. As a byproduct, we released the codes, and involved parameters to benefit other researchers.
Framework

Code
Download (updated 10/22/2019)
You can also find the detailed pytorch version from our github. (updated 11/22/2020)
Copyright (C) <2018> Shandong University
This program is licensed under the GNU General Public License 3.0 (https://www.gnu.org/licenses/gpl-3.0.html). Any derivative work obtained under this license must be licensed under the GNU General Public License as published by the Free Software Foundation, either Version 3 of the License, or (at your option) any later version, if this derivative work is distributed to a third party.
The copyright for the program is owned by Shandong University. For commercial projects that require the ability to distribute the code of this program as part of a program that cannot be distributed under the GNU General Public License, please contact <hanxianjing2018@gmail.com> to purchase a commercial license.